Many of our readers no doubt already understand the immense importance of data analysis within the contemporary business landscape.
Wide-scale data collection has become completely normalized, and there are new means of collecting various types of valuable data.
Data itself is assumed to be valuable, and it is, but in practice, that value needs to be revealed via the process of data analysis.
With relevant context and analysis, data becomes valuable information, but given the truly incredible amounts of data that are being collected by various businesses and organizations, it would be nearly impossible for data analysts to analyze all available data in an accurate and efficient fashion.
But as is the case in so many other areas of professional life, emerging technologies are streamlining data analysis, which means that larger and larger data sets can now be analyzed with greater accuracy.
But this is only a cursory overview of data analysis in the modern day. For details and further discussion of specific emerging technologies that are poised to change the data analysis game, we turn to data analyst and researcher Ravindra Patel.
With multiple post-graduate degrees in hand, Patel has built a successful career in data analysis and research, staying up-to-date on the technologies that are making waves in data analysis right now and looking forward to the inevitable future changes.
With Patel’s assistance, this article will provide brief overviews of some of the most exciting technologies in the data analysis space, including digital threads, data catalogs, Big Data, data intelligent software, AI machine learning, relational databases, and AutoML.
But first, an explanation of data analysis’ wide-ranging utility.
The utility of data analysis
In case there was any doubt about the usefulness of data analysis across multiple industries, Patel explained just how advanced data analysis has become and how it helps businesses to analyze information and obtain valuable insights.
Also, this can all be accomplished more quickly than ever before thanks to the integration of some of the technologies we’ll be talking about momentarily, including, in particular, machine learning.
“Data analysis has lots of features that can help an organization to grow its revenue by making the right decision through analyzing data. Charts, maps, and diagrams can be used to interpret and present data. In short, data science and machine learning are using a predictive method to create a simple system and work smartly to achieve a goal with less effort and optimal performance.”
So the goal, it would seem, is to intelligently predict future conditions to enable more agile business decisions.
But how is all of this made possible? That’s right, it’s time to dive into the details. Here are some of the most significant technologies shaking up data analysis right now.
Digital threads
One of the major challenges of data analysis today is managing the large amounts of data that have already been collected and finding ways to access relevant data precisely when it’s needed.
Digital threads are making that much easier, though explaining exactly how they work is difficult. Rather, digital threads are best understood in practice.
Digital threads are a way of organizing all data relevant to a specific entity or asset.
Patel described digital threads like this:
“A digital thread construct is a live, integrated repository and audit trail of real-time data generated across the end-to-end lifecycle of data entities such as products, employees, and customers.”
Otherwise, one of these entities, like a product, might generate data that gets spread out across different functions or areas. Digital threads make it far easier to access the right data about one of these entities at the right time.
These threads are one type of tool that makes it easier to organize the vast amounts of data already collected.
Data catalogs
Continuing in the area of organizing data, data catalogs are another key technology in data analysis.
As you can tell from the name, data catalogs are based around the idea of a catalog, a complete record of all entries in a specific category.
Comprehensive mail-order catalogs of bygone decades listed a store’s entire available inventory, and now data catalogs use metadata to provide views of available data.
Once again, this makes it far easier to find data when it’s needed.
As Patel explains below, these catalogs are currently popular with different types of users.
“Data catalogs, which provide centralized views, via metadata, of data assets across enterprises, are emerging as competitive tools used by data managers and business users alike.”
Beyond the basic organization and management of data, data catalogs can also allow individual users or groups of users to collect, access, and enrich metadata which can then aid in the processes of data discovery and data governance.
Data intelligence software
The next game-changer is something called data intelligence software, and it requires a brief explanation of the underlying concept.
Data intelligence, on its own, refers to using artificial intelligence (AI) and machine learning (ML) to analyze data and transform it into what are called intelligent data insights.
Intelligent data insights are, in other words, lessons learned from existing data once it has been analyzed.
Data intelligence software, then, is software that supports data intelligence. It’s software that makes it easier to utilize data-driven decision-making.
Patel elaborates:
“Data intelligence software provides a flexible, automated framework that enables the identification, understanding, control of, and insights from your data estate, facilitating enterprise data coordination.”
Data intelligence software is a significant step forward for data analysis because it offers a ready-made solution to those who otherwise wouldn’t know how to use AI and ML to analyze their data.
A common example given elsewhere online is how CRM software (customer relationship management) software steadily became commonplace for many public-facing businesses.
In a similar fashion, data intelligence software, or DI software, is becoming integrated into many businesses, as it makes data analysis much simpler and easier for companies still relatively unacquainted with Big Data.
AI machine learning
The final technology we’d like to discuss here is more abstract and versatile than any of the others mentioned here.
Even if you aren’t involved directly with Big Data in your career, you have most likely still heard the terms artificial intelligence and machine learning thrown around quite a lot in the past twenty years.
Here, we’re specifically talking about machine learning that is powered by AI technology. More specifically still, we’re referring to ML tech that is applied directly to the field of data analysis.
An idea that we’ve returned to again and again in this article is how collected data is too vast and complex for humans to effectively analyze on their own.
Could individual data analysts, in theory, analyze vast amounts of data without the assistance of advanced technology? Yes, but the process would take so long that the results would likely be outdated, and there would be a much higher chance of encountering errors.
That’s exactly why automation is the name of the game here, and AI-driven machine learning is an especially exciting frontier.
Machine learning functions could potentially solve many problems and even further streamline the data analysis processes currently being used.
Patel confirms just how important AI machine learning is and will continue to be in the years to come.
“Al machine learning data functions will be profound, especially if an enterprise seeks to use data-driven decision-making. A data analyst uses the data from these game-changing technologies to generate meaningful insights for better decision-making.”
That’s the ultimate goal of successful data analysis, of course: making intelligent decisions based on information that’s verified by hard data.
We definitely haven’t seen the full extent of what machine learning can offer to not only data analysis but many other areas of business as well, which is a major reason behind the palpable excitement that many feel for machine learning these days.
In summary
This has been only a brief look at some of the technologies that are currently changing the data analysis field and which promise to lead to even more exciting changes in the future.
Aside from the most immediate lesson, that technology can improve the speed and accuracy of data analysis many times over, there’s a lot to learn from current data analysis technologies.
Perhaps most compelling of all is the way in which many of these technologies have made advanced data analysis accessible to many more businesses and individuals.
Just a few years ago, data analysis was much more expensive and exclusive, but that’s changing.
Data-driven decision-making seems poised to become the norm for many businesses and organizations, and by all counts, that’s a positive evolution of current decision-making norms, and both businesses and their customers stand to benefit.
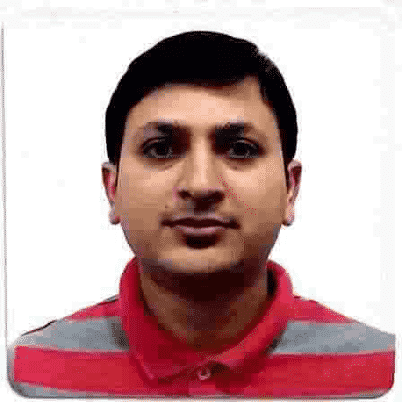
Thank you for joining us and special thanks to Ravindra Patel for his assistance and expertise.