Data mining is a hot topic, to say the very least. To the disappointment of many tech professionals, data mining has taken on a generalized, negative connotation among the general public.
The term seems to conjure images of private data being squirreled away for the sake of profit following an almost performative request for the user’s consent.
As our readers may already know, this isn’t an accurate impression of data mining in action, and it’s certainly not representative of the full breadth of data mining and its many applications.
Today’s piece is centered on data mining efforts in the healthcare industry. More specifically, we’re here to discuss the value and applications of sequential mining in healthcare. Joining us for the discussion is Alpa Reshamwala, an expert in data mining and computer engineering, who has worked in medical billing and coding with a number of prestigious institutions such as Mount Sinai Health Systems and Columbia.
Reshamwala has been using sequential mining (defined below) within various healthcare applications, and these applications give us insight into the future of healthcare, a future where patients can make intelligent decisions about treatment for themselves or loved ones, armed with the knowledge of the likely outcomes of all possible courses of treatment.
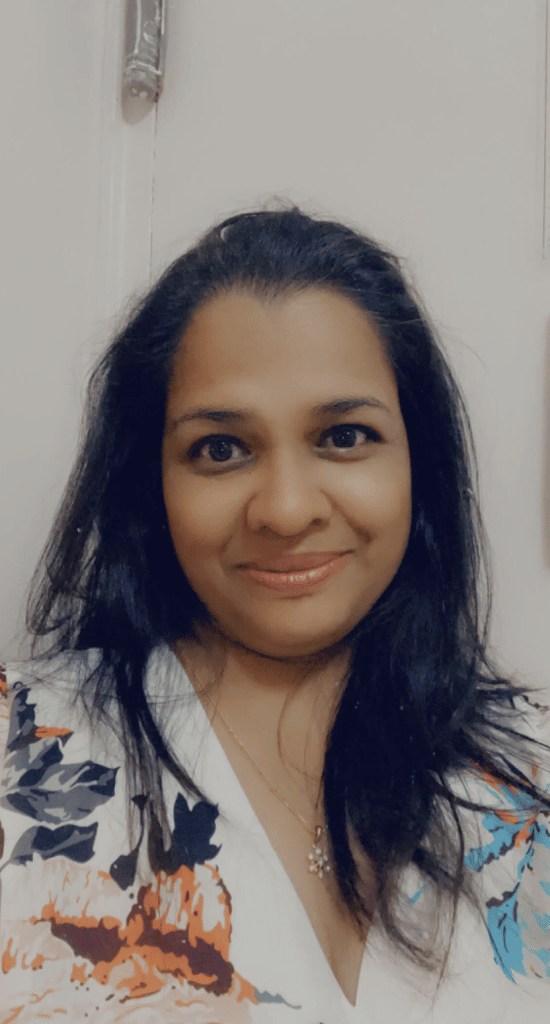
What is sequential mining?
The first point of order is to define sequential mining so that we can better understand its utility.
Here’s Reshamwala’s breakdown of sequential mining as well as sequence pattern mining:
“Sequential mining is mining for sequences or patterns in large amounts of data. Sequences are time-based events. So sequence pattern mining is the mining of similar time-based events.”
In other words, rather than searching for recurring data points, sequential mining is about looking for the repetition of entire sequences.
Identifying and analyzing these sequences can be extremely useful, especially in the realm of healthcare, where there are strong ties between health conditions, treatment, and medication.
With that in mind, let’s examine some of the most common and useful applications of sequential mining within the healthcare industry.
Sequential mining applications
Sequential mining can be applied in various ways that benefit just about everyone in the healthcare orbit, from patients themselves to healthcare institutions and even insurance providers.
But as Reshamwala explained, all of these applications and benefits stem from a singular, holistic goal.
“The main goal of data mining in healthcare is to improve the quality of care provided. This is made possible by making the computer learn from previous cases and using this intelligence to make future patient care decisions.”
Providing highly personalized care represents a substantial challenge. Healthcare providers have to care for large numbers of patients, and each patient has their own individual needs and list of pre-existing conditions.
This isn’t to say that human care teams aren’t capable of providing excellent care; they absolutely are, but adding the incredible capabilities of a digital system enhances the level of care.
For example, the computer system can be taught a patient’s symptoms, the causes of their various illnesses, and various courses of treatment. Based on all this information, the system can then select the most effective course of treatment.
“Data mining can also be applicable to drug dosage and which drugs should and should not be administered, depending on the illness. It’s also possible to recommend drugs with the patient’s pre-existing conditions in mind.”
Beyond patient care, sequential mining can also be extremely useful to insurance carriers, since it can help find the best coverage for a patient, given their illness.
“Overall, data mining enhances clinical decision making, increases diagnosis accuracy, improves patient care, avoids drugs as per illness, improves customer relationships through electronic health record systems, and many more aspects of treatment and coverage as well.”
Sequential mining, and data mining in general, are still relatively new approaches, especially within healthcare, but the advances have been significant.
In terms of current data mining innovations in the healthcare industry, Reshamwala told us that there’s a great deal of ongoing research into data mining applications for COVID-19 patient care, as well as optimized treatment plans for cancer patients.
Collected healthcare data is also being used to predict care for diabetic and heart condition patients.
As for what this mining work looks like in action for Reshamwala, here are some of the key elements.
“My work on sequential mining is, firstly, generating or mining sequences from the data. Secondly, I mine for frequent sequence occurrence in large data. From there, I add fixed as well as flexible time intervals between sequences. This results in high prediction accuracy.”
These various types of data mining can play a critical role in improving the efficiency and performance of processes in the healthcare industry.
But this work is much easier said than done. Let’s talk about some of the challenges of data mining in healthcare and beyond.
Knowledge extraction
When asked about some of the more significant challenges that she’s faced throughout her data mining work, Reshamwala discussed the complexity of what’s known as intelligence extraction or knowledge extraction.
One of the major goals of data mining, specifically within healthcare, is to predict the likely outcomes of various scenarios and courses of action.
Thankfully, Reshamwala has previously gained experience in predicting outcomes based on data when she worked as an Assistant Professor of Computer Engineering. Specifically, she was able to predict at-risk students based on test score data.
Of course, when predicting healthcare outcomes, the stakes are much greater, and as she describes here, this work is best left to experts.
“To predict healthcare-related data, you need a thorough knowledge of the raw data to extract intelligence, as healthcare data contains some very crucial information.”
But the benefits of extracting knowledge from healthcare data are enormous, as we discussed earlier.
The challenge inherent to knowledge extraction also reinforces the need for human involvement in the process. Data mining, even sequential mining, isn’t an automatic solution, and especially where treatment is concerned, there need to be experts involved who can check and double-check results.
Patient care decisions
Before closing out the article, it’s worth talking in more detail about how widespread implementation of sequential mining could impact the future of healthcare, especially with regard to patients.
Anyone who has faced a serious illness themselves or cared for a loved one diagnosed with a serious illness knows how intimidating and overwhelming it can be to navigate the early stages of treatment.
Patients have to sort through a large amount of information and consider many different treatment options. But of course, many treatment plans are also fraught with uncertainty.
Chances of success are different for each patient, and in the past, doctors and other healthcare professionals could only make educated guesses as to how a patient will respond to treatment.
There are many factors that can affect a patient’s response to treatment, including age, pre-existing conditions, overall health, and other medications they happen to be taking.
These are life-changing decisions, and many patients make these decisions largely in the dark.
Data mining represents a major shift in patient care and treatment decision-making, and this shift consists of finding other similar patients and scenarios and learning from them.
As more data is collected and analyzed over time, the better the chances are of being able to find a precedent for the specifics of a patient’s situation.
Reshamwala does an excellent job of summarizing the positive impact of healthcare data mining in the following quote:
“These data mining outcomes can help a patient make the right care decisions for their illness or condition. They can be made aware of the post-cure or subsequent conditions that we’ve learned about from similar previous cases and can plan for further courses of treatment. Patients can also look for the best possible insurance plan for their situation. Through the use of data mining, patient care will be at its highest level.”
This isn’t just theory. These outcomes are real, and at a basic level, they’re all about making better use of medical records.
The applications of these advanced data mining techniques offer opportunities for healthcare providers to be better, for insurance carriers to be better, and for patients to make better choices for the well-being of themselves and their loved ones.